PhD Defence: Gertjan van den Burg
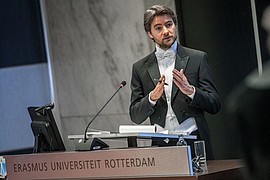
In his dissertation ‘Algorithms for Multiclass Classification and Regularized Regression’, Erim’s Gertjan van den Burg presents several new algorithms for both multiclass classification and regularized regression problems.
Gertjan defended his dissertation in the Senate Hall at Erasmus University Rotterdam on Friday, 12 January 2017 at 9:30. His supervisor was Prof. Patrick Groenen and his co-supervisor was Dr Andreas Alfons. Other members of the Doctoral Committee are Prof. Dennis Fok (EUR), Prof. Alfred Hero (University of Michigan), Prof. Henk Kiers (University of Groeningen), Prof. Arthur Tenenhaus (CentraleSupelec), Prof. Cajo ter Braak (Wageningen University & Research), Prof. André Martins (Instituto Superior Tecnico).
About Gertjan van den Burg
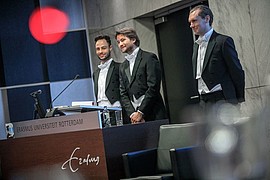
Gerrit Jan Johannes (Gertjan) van den Burg grew up in the greenhouse-packed area of Westland, The Netherlands. In 2006 he started the BSc program in Applied Physics at Delft University of Technology. During his studies he joined the pre-master program in Econometrics at Erasmus University Rotterdam. Between 2009 and 2012 he completed the MSc degree in Applied Physics at Delft University of Technology and the MSc degree in Econometrics and Management Science at the Erasmus University Rotterdam. Gertjan joined ERIM and the Econometric Institute in December 2012.
Gertjan's research focuses on the development of machine learning algorithms, particularly for multiclass classification and regularized regression problems. His research addresses the foundational aspects of machine learning techniques such as loss functions and optimization algorithms, as well as the practical aspects such as algorithm implementation and benchmarking. Gertjan has presented his work at various international conferences and has visited the University of Michigan to collaborate on research. One chapter of his dissertation has been published in the Journal of Machine Learning Research whereas another chapter is currently under review at a top machine learning journal.
Gertjan also has significant experience in teaching. During his PhD he was a teaching assistant and thesis supervisor at the BSc and MSc levels and a lecturer for the Matlab module in a BSc programming course. Gertjan pioneered the use of automatically graded programming exercises at the Erasmus School of Economics, for which he received the 2016 Top Educator Award. For more about Gertjan, visit https://gertjanvandenburg.com.
Thesis Abstract
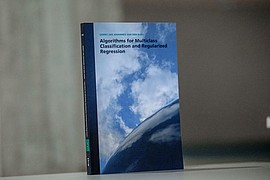
Multiclass classification and regularized regression problems are very common in modern statistical and machine learning applications. Multiclass classification problems require the prediction of class labels: given observations of objects that belong to certain classes, can we predict to which class a new object belongs? The regularized regression problem on the other hand is a variation of a common technique that measures how changes in independent variables influence an observed outcome. In regularized regression constraints are placed on the coefficients of the regression model to enforce certain properties in the solution, such as sparsity or limited size. In this dissertation several new algorithms are presented for both multiclass classification and regularized regression problems.
For multiclass classification an algorithm is presented that extends the binary support vector machine in a general way, while maintaining competitive performance and training time. Furthermore, accurate estimates of the Bayes error are applied to both meta-learning and the construction of so-called classification hierarchies: structures that decompose a multiclass classification into several binary classification problems.
For regularized regression problems a general algorithm is presented for problems where the regularization function is a measure of the size of the coefficients. In the proposed algorithm the nonconvexity in the problem is slowly introduced while iterating towards a solution. The empirical performance and theoretical convergence properties of the algorithm are analysed with numerical experiments that demonstrate that the algorithm can obtain globally optimal solutions.
Photos: Chris Gorzeman / Capital Images