PhD Defence: Ronald de Vlaming
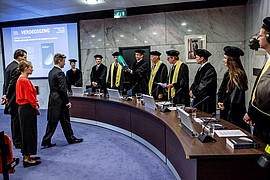
In his dissertation ‘Linear Mixed Models in Statistical Genetics’ ERIM’s Ronald de Vlaming investigates several aspects of LMMs and related methods, such as ridge regression and LD-score regression.
Ronald defended his dissertation in the Senate Hall at Erasmus University Rotterdam on Thursday, 6 July 2017 at 15:30. His supervisors were Prof. Roy Thurik, Prof. Patrick Groenen and Prof. Philipp Koellinger. Other members of the Doctoral Committee are Prof. Richard Paap (Erasmus School of Economics), Prof. Danielle Posthuma (VU University Amsterdam), Dr. Katrijn Van Deun (Tilburg University), Dr. Jonathan Beauchamp (University of Toronto), and Dr. Matthew Keller (University of Colorado, Boulder).
About Ronald de Vlaming
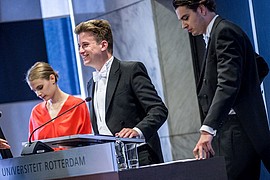
Ronald de Vlaming (1987) holds a M.Sc. degree cum laude in Econometrics and Management Science from the Erasmus School of Economics (ESE), Erasmus University Rotterdam. In 2013 Ronald started as a Ph.D. candidate under the supervision of professors A. Roy Thurik, Patrick J. F. Groenen, and Philipp D. Koellinger. He carried out his research within the Department of Applied Economics at the ESE as a member of the Erasmus University Rotterdam Institute for Behavior and Biology and of the Social Science Genetic Association Consortium. In 2016 Ronald visited the Institute for Molecular Bioscience at the University of Queensland for a period of three months. During his research visit he was supervised by professor Peter M. Visscher.
Ronald’s research focuses on the application of statistical methods, matrix algebra, and numerical methods to estimation problems that arise in the study of the genetic architecture of complex traits. His work has been published in the following peer-reviewed journals: PLOS Genetics, BioMed Research International, Nature Genetics, and Nature. He has presented his work at meetings of the Behavior Genetics Association, the Conference on Computational Statistics, and the European Human Genetics Conference. Ronald will continue his career as postdoctoral researcher at the VU University Amsterdam
Thesis Abstract
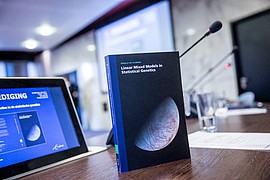
One of the goals of statistical genetics is to elucidate the genetic architecture of phenotypes (i.e., observable individual characteristics) that are affected by many genetic variants (e.g., single-nucleotide polymorphisms; SNPs). A particular aim is to identify specific SNPs that are robustly associated with a given phenotype using a so-called genome-wide association study (GWAS).
Although GWAS sample sizes have increased in recent years, the number of SNPs still tends to vastly exceed sample sizes. Hence, multiple regression cannot be used to infer the association between SNPs and a phenotype jointly. Instead, the linear mixed model (LMM) has become a popular tool in statistical genetics. By placing a reasonable prior on SNP effects, LMMs can be used to jointly estimate SNP effects and to infer their contribution to phenotypic variance.
In this dissertation, I investigate several aspects of LMMs and related methods, such as ridge regression and LD-score regression. In addition, an LMM is used to develop an online tool, called MetaGAP, which quantifies the statistical power of a GWAS in case of heterogeneity in underlying subsamples. Using MetaGAP, I show that ongoing GWAS efforts are well-powered even for considerably heterogeneous phenotypes. This prediction is bolstered by a GWAS of reproductive choices, reported here, that finds twelve robustly associated SNPs.
I conclude that current GWAS sample sizes enable researchers to uncover parts of the genetic architecture of complex social-scientific outcomes and posit that GWAS efforts will soon attain sufficient predictive accuracy for useful applications throughout the social sciences.
Photos: Chris Gorzeman / Capital Images