PhD Defence: Bruno Jacobs
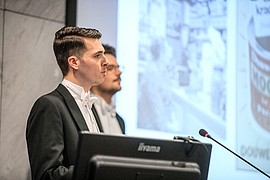
In his dissertation ‘Marketing Analytics for High-Dimensional Assortments’ ERIM’s Bruno Jacobs presents model-based methods that can be used to model purchase decisions in high dimensional product assortments.
Bruno defended his dissertation in the Senate Hall at Erasmus University Rotterdam on Friday, 22 December 2017 at 13:30. His supervisors were Prof. Bas Donkers and Prof. Dennis Fok. Other members of the Doctoral Committee are Prof. Tammo Bijmolt (University of Groningen), Prof. Benedict Dellaert (EUR), Prof. Philip Hans Franses (EUR), Prof. Richard Paap (EUR), and Dr Jason Roos (EUR).
About Bruno Jacobs
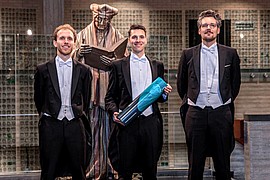
Bruno Jacobs (1988) holds a Master's degree in Econometrics and Management Science from Erasmus University Rotterdam. In 2012 he joined the Erasmus Research Institute of Management (ERIM) as a Ph.D. student under the supervision of Prof. Bas Donkers and Prof. Dennis Fok. He carried out his research within the Marketing department and the Econometric Institute at the Erasmus School of Economics. In 2015, Bruno was a visiting scholar at Columbia University in the City of New York, hosted by Prof. Asim Ansari. Bruno currently works as an Assistant Professor in Marketing at the Robert H. Smith School of Business, University of Maryland, College Park.
Bruno's research is situated at the intersection of marketing, machine learning, and econometrics. Currently, he focuses on the development of new methods to describe, understand, and predict customer decisions in high-dimensional product assortments. Scalability is one of the focal points in his research. For his research Bruno worked with national and international retailers. His work has been published in Marketing Science and presented at the Wharton School, multiple INFORMS Marketing Science Conferences, and a Marketing Dynamics Conference.
Thesis Abstract
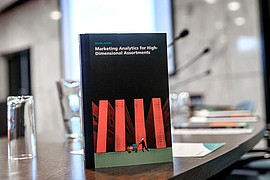
Over the past two decades online retailing has become ubiquitous and today’s large online retailers enable customers to purchase virtually any product. As a consequence product assortments at such retailers are of a different order of magnitude compared to the traditional brick-and-mortar stores. In this dissertation model-based methods are presented that can be used to model purchase decisions in such high-dimensional product assortments. These methods are able to accurately predict at the individual customer level which product will be purchased next out of the large assortment. In addition, the methods provide substantive insights in the patterns that underlie the observed purchase behavior. The applicability of such methods in practice hinges on their scalability and this holds especially true for online retailers. Model results should be rapidly obtained and the estimation time should not significantly increase in case the customer base or product assortment expands. Scalability is therefore a focal point in this dissertation. The methods introduced are adaptations and extensions of fast scalable methods from the machine learning literature that make these methods also suitable for the online retailing context. This ensures that estimation times remain feasible even if the size of the retailer increases and opens the way for advanced model-based marketing analytics in high-dimensional assortments.
Photos: Chris Gorzeman / Capital Images